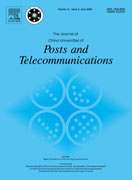
★ EI核心期刊
(双月刊, 1994年创刊)
主办单位:北京邮电大学
编委会主任:乔建永
主 编:张平
国际标准刊号:ISSN 1005-8885
国内统一刊号:CN 11-3486/TN
邮发代号:2-629
王昊天 刘立洲 邱玲 张磊
中国邮电高校学报(英文), 2025, 32 (1). doi: 10.19682/j.cnki.1005-8885.2025.0005
刘雯 任海龙 邓中亮
中国邮电高校学报(英文), 2025, 32 (1). doi: 10.19682/j.cnki.1005-8885.2025.0006
张双根 于涛 王玉兰 周冉 程志华
中国邮电高校学报(英文), 2025, 32 (1). doi: 10.19682/j.cnki.1005-8885.2025.0007
吴进 苏正东 王辉立 苏泽林
中国邮电高校学报(英文), 2025, 32 (1). doi: 10.19682/j.cnki.1005-8885.2025.0003
张玺君 李喆
中国邮电高校学报(英文), 2025, 32 (1). doi: 10.19682/j.cnki.1005-8885.2025.0001
张玺君 崔勇 张红 夏子尧
中国邮电高校学报(英文), 2025, 32 (1). doi: 10.19682/j.cnki.1005-8885.2025.0002
山蕊 许佳宁 霍紫晴
中国邮电高校学报(英文), 2025, 32 (1). doi: 10.19682/j.cnki.1005-8885.2025.0004
刘兆函 韩宇南 Qiu Xianbo 李贤丽
中国邮电高校学报(英文), 2025, 32 (1). doi: 10.19682/j.cnki.1005-8885.2025.0008
2024, Vol.31 | No.5 | No.4 | No.3 | No.2 | No.1 | |
---|---|---|---|---|---|---|
2023, Vol.30 | No.6 | No.5 | No.4 | No.3 | No.2 | No.1 |
2022, Vol.29 | No.6 | No.5 | No.4 | No.3 | No.2 | No.1 |
2021, Vol.28 | No.6 | No.5 | No.4 | No.3 | No.2 | No.1 |
2020, Vol.27 | No.6 | No.5 | No.4 | No.3 | No.2 | No.1 |
2019, Vol.26 | No.6 | No.5 | No.4 | No.3 | No.2 | No.1 |
2018, Vol.25 | No.6 | No.5 | No.4 | No.3 | No.2 | No.1 |
2017, Vol.24 | No.6 | No.5 | No.4 | No.3 | No.2 | No.1 |
2016, Vol.23 | No.6 | No.5 | No.4 | No.3 | No.2 | No.1 |
2015, Vol.22 | No.6 | No.5 | No.4 | No.3 | No.2 | No.1 |
2014, Vol.21 | No.6 | No.5 | No.4 | No.3 | No.2 | No.1 |
2013, Vol.20 | No.6 | No.5 | No.4 | No.3 | No.2 | No.1 |
2012, Vol.19 | No.6 | No.5 | No.4 | No.3 | No.2 | No.1 |
2011, Vol.18 | No.6 | No.5 | No.4 | No.3 | No.2 | No.1 |
2010, Vol.17 | No.6 | No.5 | No.4 | No.3 | No.2 | No.1 |
2009, Vol.16 | No.6 | No.5 | No.4 | No.3 | No.2 | No.1 |
2008, Vol.15 | No.4 | No.3 | No.2 | No.1 | ||
2007, Vol.14 | No.4 | No.3 | No.2 | No.1 | ||
2006, Vol.13 | No.4 | No.2 | No.3 | No.1 |
» | Graph convolutional network combined with random walks and graph attention network for node classification |
陈勇 谢小竹 翁伟 | |
2024 Vol. 31 (3): 1-14 |
» | Black-box membership inference attacks based on shadow model |
Han Zhen, Zhou Wen'an, Han Xiaoxuan, Wu Jie | |
2024 Vol. 31 (4): 1-16 |
» | Personalized trajectory data perturbation algorithm based on quadtree indexing |
刘琨 靳军辉 王辉 申自浩 刘沛骞 | |
2024 Vol. 31 (4): 17-27 |
» | Artificial rabbit optimization algorithm based on chaotic mapping and Levy flight improvement |
吴进 苏正东 高亚琼 冯浩然 | |
2024 Vol. 31 (4): 54-69 |
» | Improving Link Prediction Models through a Performance Enhancement Scheme: A Study on Semi-Supervised Learning and Model Soup |
亓东林 陈曙东 杜蓉 佟达 余泳 | |
2024 Vol. 31 (4): 43-53 |
» | Power and Rate Adaption in Wireless Communication Systems with Energy Harvesting–Based on Soft Decision Decoding |
雷维嘉 于顺洪 刘美玎 | |
2024 Vol. 31 (4): 70-82 |
» | Dynamic coverage of mobile multi-target in sensor networks based on virtual force |
黄庆东 王梅 韩壮 陈晨 | |
2024 Vol. 31 (4): 83-94 |
» | Deep kernel extreme learning machine classifier based on the improved sparrow search algorithm |
赵广元 雷渝 | |
2024 Vol. 31 (3): 15-29 |
» | CNN demodulation model with cascade parallel crossing for CPM signals |
杨嘉晨 段瑞枫 李诚驹 | |
2024 Vol. 31 (3): 30-42 |
» | Used car price prediction based on XGBoost and retention rate |
沈雨田 陈建 戴敏 张思瑞 徐晶 王青 | |
2024 Vol. 31 (3): 72-79 |
» | W-band millimeter wave vialess microstrip-to-microstrip vertical transition in multilayer LCP substrate |
刘维红 张旭 关东阳 | |
2024 Vol. 31 (3): 87-94 |
» | LRChain: Data protection and sharing method of learning archives based on consortium blockchain |
兰丽娜 高语晗 石瑞生 吴芬芬 | |
2024 Vol. 31 (4): 28-42 |
» | Compact multilayer liquid crystal polymer lowpass filter with 8-shaped inductor |
刘维红 王国秀 刘清冉 | |
2024 Vol. 31 (3): 80-86 |
» | Bidirectional position attention lightweight network for massive MIMO CSI feedback |
李军 王昱凯 张志晨 何波 郑文静 林霏 | |
2024 Vol. 31 (5): 1-11 |
» | Fine-grained emotion prediction for movie and television scene images |
苏志斌 周璇烨 刘冰 任慧 | |
2024 Vol. 31 (3): 43-55 |
» | Fast Fourier transform convolutional neural network accelerator based on overlap addition |
游晨 李德建 冯曦 沈冲飞 魏继增 刘昱 | |
2024 Vol. 31 (5): 71-84 |
» | Design of graph computing accelerator based on reconfigurable PE array |
邓军勇 贾彦亭 张宝祥 康钰春 鲁松涛 | |
2024 Vol. 31 (5): 49-63 |
» |
Naive-LSTM enabled service identification of edge computing in power Internet of things |
白晖峰 霍超 张港红 尹志斌 | |
2024 Vol. 31 (5): 34-41 |
» | Recognition of LPI radar signal based on dual efficient network |
李辉 秦怡博 侯庆华 程远洋 | |
2024 Vol. 31 (5): 12-22 |
» | IHMP: an improved hierarchical motion planner for mobile manipulator in static environment |
王斌鹏 黄后钦 徐舫舟 鞠殿元 李泽强 冯超 | |
2024 Vol. 31 (3): 56-71 |
» | Remaining useful life prediction of lithium-ion batteries using a fusion method based on Wasserstein GAN |
周温丁 鲍士兼 许方敏 赵成林 | |
2020 Vol. 27 (1): 1-9 |
» | IDL: Evaluating software quality based on PageRank algorithm |
周国强, 范译, ZHANG Shuai WANG Yilun DAI Gui-lan | |
2020 Vol. 27 (1): 10-25 |
» | Analysis of 3D NAND technologies and comparison between charge-trap-based and floating-gate-based flash devices |
刘世军 邹雪城 | |
2017 Vol. 24 (3): 75-82 |
» | Dense small cell clustering based on undirected weighted graph for local mobility management |
李营营 马忠贵 刘立宇 闫文博 | |
2016 Vol. 23 (5): 32-39 |
» | Mobile node aware opportunistic routing in dynamic wireless sensor network |
吕晓军 贾新春 韩宗源 杨波 郝军 | |
2016 Vol. 23 (5): 15-25 |
» | Research on intelligent fault diagnosis based on time series analysis algorithm |
陈刚;刘洋;周文安;宋俊德 | |
2008 Vol. 15 (1): 68-74 |
» | Novel high-PSRR high-order curvature-compensated bandgap voltage reference |
周前能 闫凯 林金朝 庞宇 李国权 罗伟 | |
2016 Vol. 23 (2): 66-72 |
» | Fog computing for vehicular Ad-hoc networks: paradigms, scenarios, and issues |
康慨 王枞 LuoTao | |
2016 Vol. 23 (2): 56-65 |
» | Fine-grained analysis of cellular smartphone usage characteristics based on massive network traffic |
Gui Xiaolin, Liu Jun, Li Chenyu, Lü Qiujian, Lei Zhenming | |
2016 Vol. 23 (3): 70-75 |
» | Construction of authentication codes with distrust arbitration from polynomials over finite fields |
Chen Shangdi, Tian Wenjing, Li Xue | |
2017 Vol. 24 (1): 87-95 |
» | Mining microblog user interests based on TextRank with TF-IDF factor |
屠守中 黄民烈 | |
2016 Vol. 23 (5): 40-46 |
» | Autonomous parking control for intelligent vehicles based on a novel algorithm |
高洪波 XieGuotao 张新钰 ChengBo | |
2017 Vol. 24 (4): 51-56 |
» | Measuring web page complexity by analyzing TCP flows and HTTP headers |
Cheng Weiqing,Hu Yangyang, Yin Qiaofeng, Chen Jiajia | |
2017 Vol. 24 (6): 1-13 |
» | EVM simulation and analysis in digital transmitter |
谭晓衡,李腾蛟 | |
2009 Vol. 16 (6): 43-48 |
» | LPC parameters substitution for speech information hiding |
吴志军,高伟,杨伟 | |
2009 Vol. 16 (6): 103-112 |
» | Non-orthogonal pilot pattern for sparse channel estimation in large-scale MIMO-OFDM system |
徐超 张建华 尹长川 | |
2016 Vol. 23 (4): 63-68 |
» | Design of hexagon microstrip antenna for vehicle-to-vehicle communication |
郝宏刚 李佳玉 Huang Daili Luo Wei | |
2016 Vol. 23 (4): 69-76 |
» | Reduced frame set on wireless distorted video for quality assessment |
邵华 温向明 路兆铭 陈亚文 陆镜宇 | |
2016 Vol. 23 (4): 77-82 |
» | Resource Allocation Scheme Based on Game Theory in Heterogeneous Networks |
Wu Yalong, Li Xi, Zhang Heli, Wang Ke | |
2016 Vol. 23 (3): 57-62 |
» | Efficient closed-form solution for target localization using TDOA measurements in the presence of sensor position errors |
闫雷兵 张业荣 陆音 | |
2017 Vol. 24 (4): 1-9 |
» | Energy-balanced unequal clustering protocol for wireless sensor networks |
蒋畅江 石为人 向敏 唐贤伦 | |
Acta Metallurgica Sinica(English letters). 2010 Vol. 17 (4): 94 -99 | |
被引次数: Baidu(220) |
» | Spreading behavior of SIS model with non-uniform transmission on scale-free networks |
夏承遗,刘忠信,陈增强,袁著祉 | |
Acta Metallurgica Sinica(English letters). 2009 Vol. 16 (1): 27 -31 | |
被引次数: Baidu(132) |
» | Survey of multi-channel MAC protocols for IEEE 802.11-based wireless Mesh networks |
雷宏江 高潮 郭永彩 张治中 | |
Acta Metallurgica Sinica(English letters). 2011 Vol. 18 (2): 33 -44 | |
被引次数: Baidu(122) |
» | An improved multilevel fuzzy comprehensive evaluation algorithm for security performance |
LI Ling-juan;SHEN Ling-tong | |
Acta Metallurgica Sinica(English letters). 2006 Vol. 13 (4): 48 -53 | |
被引次数: Baidu(115) |
总访问量
今日访问
在线人数